In an op-ed for The Wall Street Journal last year, Curt Levey, president of the Committee for Justice, and Ryan Hagemann, the Niskanen Center’s senior director for policy, dissected the confusing debate surrounding calls for algorithmic “transparency.” They concluded that arguments in favor of making artificial intelligence (AI) systems more transparent, though well-intentioned, were unlikely to produce the outcomes that advocates sought. Instead, they offered a solution that could better balance consumer protection and innovation: algorithmic “accountability.”
In a new report by the Center for Data Innovation (CDI), Joshua New and Daniel Castro make a similar case for prioritizing algorithmic accountability over algorithmic transparency. In the ongoing debate over how, whether, and when to regulate AI, this report pushes the conversation forward in a substantive and thoughtful manner, and adds considerable meat to the bones of Levey’s and Hagemann’s op-ed.
As defined in the CDI framework, “accountability” means that the people who are held responsible for the decisions an algorithm makes are not the software engineers who developed it (the “developers”), but those who actually deploy it (the “operators”). These operators have a duty to incorporate proper controls and best practices during the deployment of the system. Since they are ultimately in control of how an AI is deployed and used, operators, not developers, should be held primarily liable for situations in which the use of such systems results in significant consumer harm.
By contrast, “transparency” requirements – when defined at all – would inevitably require organizations to expose proprietary source-code and data that inform algorithmic decision-making. This seemingly virtuous idea has two problems. First, a machine-learning algorithm is not necessarily comprehensible even when you can inspect all its code and data. Second, the algorithms themselves are the result of significant financial investment and their exposure could lead to intellectual-property theft and a decline in future research and development.
New and Castro use the case of Google’s PageRank algorithm to demolish the argument that transparency requirements would have little to no negative effect on private companies. Search engine optimization (SEO) is already estimated to be a $65 billion industry without transparency regulations in place. Imposing new requirements on Google to reveal how its algorithms work could allow them to be gamed at a whole new level — harming consumers with lower-quality search results and ultimately reducing Google’s profitability and incentives to continue innovating.
The report also does a good job showing that in many situations, a machine-learning algorithm is only as biased as the training data it is given. For example, an algorithm will perpetuate discriminatory decisions in the judicial system if it is given a historical data set chock-full of racist human decisions. Developers need to be cognizant of which data sets are training their algorithms, and operators need to be aware of the outcomes they are optimizing.
As the authors note, in many sectors, such as housing and finance, there are already laws on the books that prohibit discrimination and disparate impact. These same laws, which are already applied to human decisions, can and should be applied to algorithmic decisions as well. There is no new technology-specific regulation needed to address harms that can already be addressed by existing rules.
While New and Castro note the prevalence of failures in human decision-making, there are powerful examples that could have been more explicitly addressed in the report. For instance, they briefly mention motor vehicle fatalities, but it would have been valuable to take a deeper dive into this case study and consider the potential costs of transparency requirements that could unintentionally delay the introduction of driverless cars. In 2016, the most recent year for which we have official data, human drivers in the United States caused more than 37,000 deaths. Do we need to wait until the algorithms used in autonomous vehicles are proven to have a zero fatality rate, or can they be deployed after showing that they are safer than the status quo? Thousands of lives hang in the balance of this question.
It also would have been useful to spend time explaining the differences among AI, machine learning, and deep learning, as these terms are also widely misused in public discussion. As the chart below from Nvidia shows, these are embedded categories. Most of the public’s fears can be more narrowly attributed to deep learning — algorithms that essentially write themselves based on input data and are often abstruse, even to their developers.
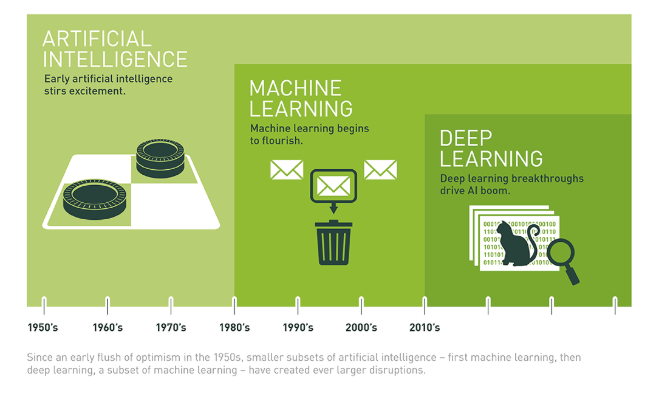
Source: Michael Copeland, “What’s the Difference Between Artificial Intelligence, Machine Learning, and Deep Learning?” Nvidia blog, 29 July 2016.
Clarifying the terminology can help steer regulatory attention to those areas of AI that may implicate significant injuries for consumers, and away from perceived or hypothetical harms that are in fact benign.
The authors also introduce a novel flowchart to show how a regulator could impose penalties on algorithm operators. In a sense, they’ve constructed what a Regulator’s Neural Network might look like when determining the existence and magnitude of an algorithmic harm. While this diagram is excellent at explaining how regulators should discipline operators after a consumer injury has been established, it is also worthwhile to consider how regulators and industry, acting separately or in collaboration, might incorporate best practices for algorithmic accountability in advance of potential harms.
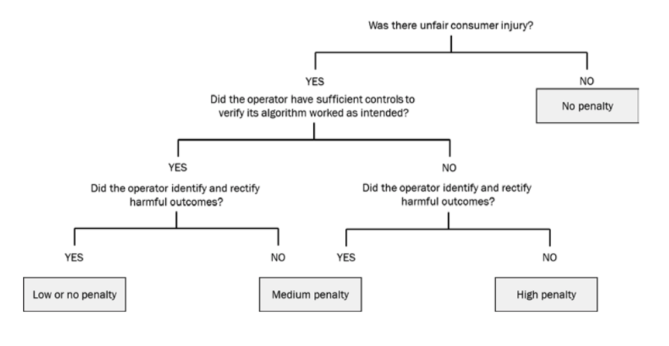
Source: Joshua New and Daniel Castro, How Policymakers Can Foster Algorithmic Accountability (Washington, D.C., Brussels: Center for Data Innovation, 21 May 2018): p. 26.
At a very high level, we could consider two important variables in algorithmic decision-making that may influence expectations for what constitutes best practices. First, the complexity of the algorithm itself. While an algorithm’s complexity isn’t necessarily a limiting factor in verifying a given outcome (after all, the outcome is simply what is observed, and doesn’t necessitate understanding the operations of the neural network or its decision-making matrix/framework), a more complicated algorithm does make it more difficult to reach an acceptable level of explainability. Second, the importance of the algorithmic decision. A highly consequential algorithmic decision almost certainly requires a heightened assurance of various accountability measures being in place, such as ensuring maximal explainability and procedural regularity.
As the box chart below shows, for unimportant decisions based on algorithms with any level of complexity, there is likely no need to impose new regulations or restrictive standards on either operators or developers. In cases where the decision is important but the algorithm is relatively simple, there may be value in providing some explanation for how the algorithm made its decision. In situations where the decision is important and the algorithm is complex, mandates for confidence measures, proof of procedural regularity, or error analysis might be necessary.
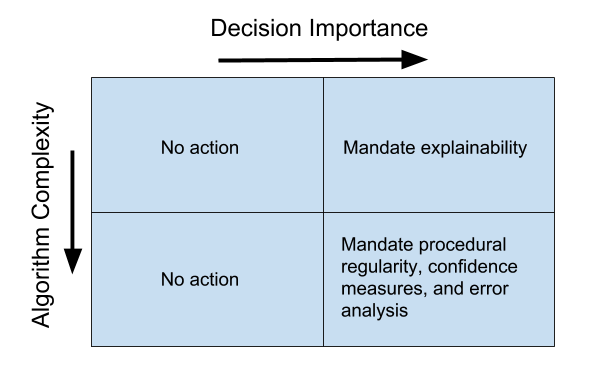
Source: Niskanen Center. (Note: This analysis is a work in progress, and feedback on both the variables and framework are more than welcome.)
As Vijay Pande, a general partner in venture capital firm Andreessen Horowitz, reminds us, “the black box in A.I. isn’t a new problem due to new tech: Human intelligence itself is — and always has been — a black box.” There are simply too many benefits of AI to stifle its development with prescriptive, technology-specific transparency requirements that we are neither able nor willing to impose on human intelligence. Narrowly-targeted accountability requirements, by contrast, will go a long way to mitigating the worst of its potential harms. In this vein, New and Castro’s report makes a compelling case for using the “innovation principle” as opposed to the “precautionary principle” when it comes to regulating algorithms.